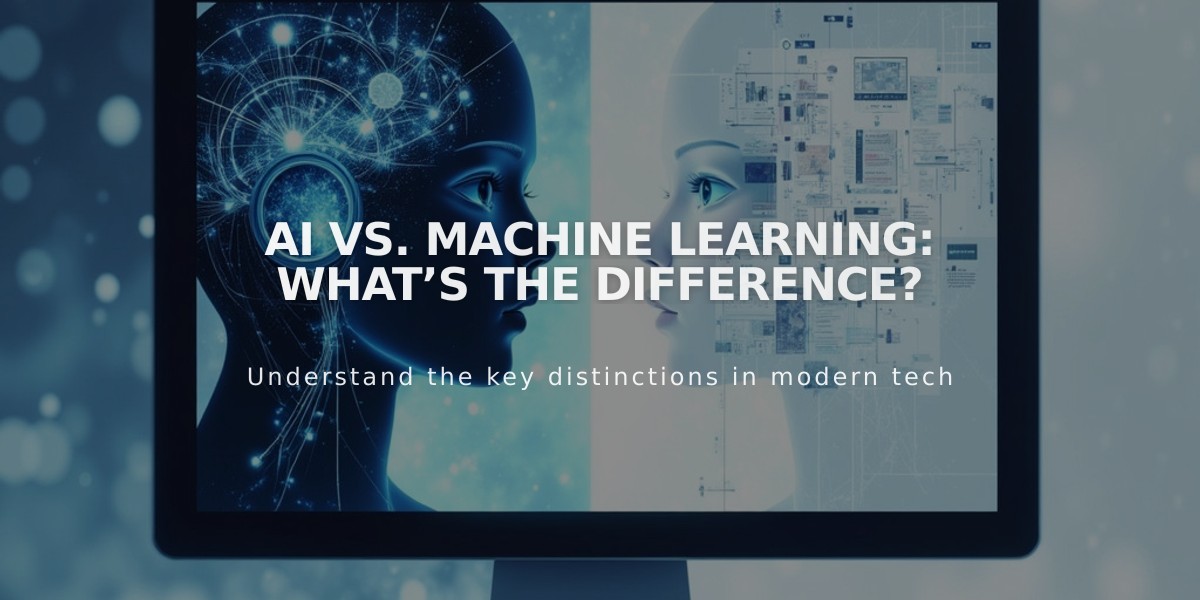
AI vs Machine Learning: Understanding the Key Differences in Today's Tech World
In today's world, artificial intelligence (AI) and machine learning (ML) are often used interchangeably. Understanding their differences is crucial for grasping their potential applications and role in driving innovation across industries.
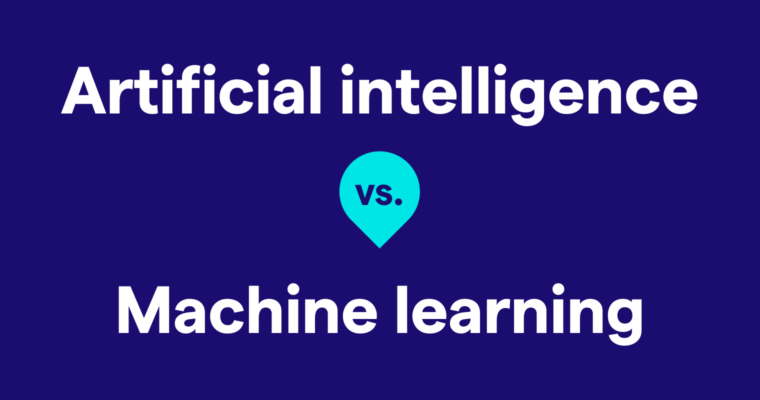
AI vs Machine Learning diagram
Artificial Intelligence (AI) replicates human mind functionality through technology. It can solve problems using algorithms and logical reasoning, with most modern systems capable of learning independently. AI ranges from simple chatbots to complex self-driving cars.
AI falls into three categories:
- Narrow AI: Specialized for specific tasks (e.g., streaming recommendations)
- General AI: Broad understanding across subjects (e.g., ChatGPT, Google Gemini)
- Superintelligent AI: Hypothetical systems exceeding human capabilities
Machine Learning (ML) is an AI subset using data and statistics to enable computer learning without explicit programming. It employs algorithms like linear regression, decision trees, and neural networks.
ML encompasses three main types:
- Supervised learning: Uses labeled data for predictions
- Unsupervised learning: Identifies patterns in unlabeled data
- Reinforcement learning: Learns through environment interaction
The key relationship: ML is a subset of AI that enables systems to learn from experience. While all ML is AI, not all AI involves machine learning.
Benefits of AI and ML:
- Enhanced efficiency through automation
- Deep data insights and pattern recognition
- Personalized user experiences
- Reduced operational costs
Challenges include:
- Heavy dependence on quality data
- Potential for bias in decision-making
- Technical complexity
- Ethical concerns regarding privacy and job displacement
Real-world applications:
- Healthcare: Disease diagnosis and treatment planning
- Banking: Fraud detection and credit scoring
- Manufacturing: Predictive maintenance and optimization
These technologies continue transforming industries through improved efficiency, accuracy, and innovation, while requiring careful consideration of ethical implications and implementation challenges.